As video consumption continues to dominate digital platforms, ensuring exceptional streaming quality has become more critical than ever. Traditional technical metrics often fall short of capturing what viewers actually perceive. That’s why Netflix developed VMAF (Video Multi-method Assessment Fusion) — a perceptual video quality metric that combines multiple evaluation methods to better reflect real human judgment. It helps content providers optimize compression and delivery without compromising the viewer experience.
What is VMAF?
Video Multi-method Assessment Fusion (VMAF) is an advanced video quality assessment algorithm developed by Netflix. Unlike traditional metrics that may evaluate isolated factors like compression artifacts or pixel-level errors, VMAF combines multiple analytical methods to measure how viewers actually perceive the overall quality of a video.
This model fuses conventional indicators such as Peak Signal-to-Noise Ratio (PSNR) and Structural Similarity Index (SSIM) to produce a more accurate and human-aligned quality score. Its purpose goes beyond simply generating a number — it’s designed to guide video encoding decisions based on real audience perception.
For example, if VMAF detects little perceived difference between two bitrate levels, a streaming provider might confidently choose the lower bitrate to save bandwidth without sacrificing viewer experience. This makes VMAF a critical tool in the streaming industry, where the challenge isn’t just delivering video efficiently, but also ensuring that content looks its best in the eyes of the audience.
Why VMAF Matters for Streaming and Video Quality Optimization
Traditional video quality metrics often fall short of reflecting how real people perceive what they watch. A video clip may score well using mathematical models like PSNR or SSIM, yet still appear blurry, blocky, or unnatural to the viewer.
This is exactly where VMAF stands out. By fusing multiple assessment methods and simulating human perception, the VMAF model produces scores that better align with real viewing experiences. It empowers:
- Streaming platforms to balance visual quality with bandwidth efficiency
- Video engineers to fine-tune encoding workflows based on perceived quality
- QA teams to enforce meaningful quality standards, not just technical ones
For example, if VMAF scores show minimal difference between two bitrate levels, providers can safely reduce bitrate to save data — without degrading viewer experience. This makes VMAF essential for maintaining high-quality streaming under real-world network conditions.
How the VMAF Model Works
The VMAF model is built on technical features and perceptual modeling. Here are its foundational elements:
1. Fusion Model
At the core is a machine learning engine trained on subjective viewer ratings. It blends various metrics into a single, perceptually meaningful output.
2. Feature Extraction
It evaluates characteristics like texture, edge clarity, and color consistency on a frame-by-frame basis.
3. Temporal Consistency
Unlike image-based methods, this approach accounts for changes over time, ensuring smooth playback and consistent quality.
4. Reference-Based Comparison
The VMAF model compares compressed video content with high-quality source material to determine the level of degradation.
How VMAF Impacts Video Processing Decisions
The VMAF model isn’t just for monitoring—it informs how content is encoded and streamed. Here’s how it’s applied:
Encoding Strategy
Content teams use these scores to adjust compression without sacrificing clarity. Higher scores indicate minimal perceived quality loss even at lower bitrates.
Automated Quality Control
The model can flag underperforming encodes, enabling scalable quality assurance processes.
Adaptive Streaming
In real-time environments, it helps optimize quality tiers for seamless switching between resolutions with minimal perceptual impact.
Competitive Advantage
Delivering better user-perceived video fidelity strengthens viewer retention and platform differentiation.
How ZEGOCLOUD Enhances VMAF-Based Video Quality Optimization
For developers building real-time video applications, platforms like ZEGOCLOUD provide tools and APIs to improve media delivery. By integrating perceptual scoring models into video pipelines, ZEGOCLOUD enables precise encoding adjustment, real-time playback analysis, and bandwidth-efficient streaming—all while centering on user experience.
Whether powering live broadcasts, virtual classrooms, or interactive video calls, ZEGOCLOUD helps teams implement perceptual metrics for more responsive and scalable delivery across devices.
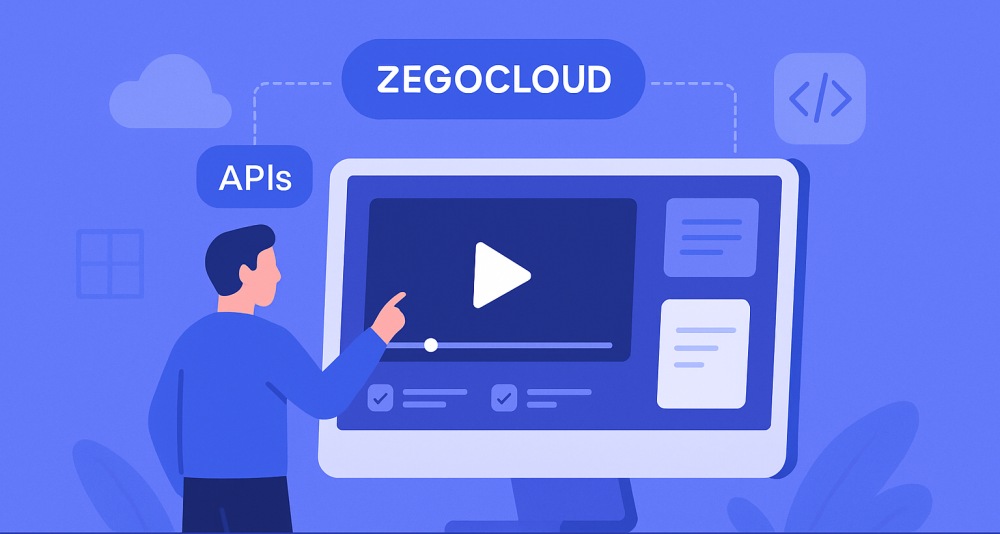
Best Practices for Using VMAF in Real-World Applications
To get the most value from perceptual video quality tools, consider the following implementation tips:
1. Adapt to Content Type
Different types of video content behave differently under compression. For example, sports and animation may require distinct encoding strategies. Tailoring your perceptual model to specific genres can improve scoring accuracy and viewer experience.
2. Pair with Other Metrics
While perceptual scores are highly informative, combining them with traditional metrics like PSNR or SSIM offers a fuller picture. This helps balance algorithmic precision with real-world perceptual impact.
3. Test Across Devices
User perception varies significantly across devices. A video that looks sharp on a smartphone might appear blurry on a large-screen TV. Running VMAF tests across multiple resolutions and screen sizes helps maintain consistent quality.
4. Target Bandwidth Constraints
In bandwidth-limited environments, it’s essential to find the optimal tradeoff between file size and perceived quality. Use quality scores to evaluate how well your video performs at lower bitrates without compromising user satisfaction.
5. Dive into Frame-Level Analysis
Instead of relying solely on the overall score, inspect how quality fluctuates throughout the video. Frame-level analysis can uncover issues like motion blur, dark scene degradation, or dropped quality during high-action sequences.
The Future of Video Quality Evaluation
As global video consumption grows and bandwidth efficiency becomes essential, perceptual quality metrics will continue gaining prominence. By uniting technical precision with human perception, tools like the VMAF model provide reliable guidance for balancing resource use and visual excellence.
Adopting such technologies empowers content providers to go beyond technical compliance — ensuring every frame not only looks good, but feels right to the viewer.
Conclusion
Perceptual video assessment has transformed the way we define and deliver video quality. With smarter scoring systems now available, developers, engineers, and content platforms can make informed decisions that enhance user experience. Whether optimizing compression or fine-tuning adaptive streams, these tools help ensure that what reaches the screen truly resonates with the viewer.
Let’s Build APP Together
Start building with real-time video, voice & chat SDK for apps today!